
The amount of data required for predictive analytics or prescriptive analytics can't be reliably measured through legacy data processing and analysis methods, let alone strictly through strict human intelligence.ĪI and ML automate data processing, analysis, and report generation, and can uncover data trends and patterns in seconds or minutes, allowing for real-time insights into critical operations. If there's one chief limitation of traditional analytics tools that AI-driven analytics models don't have, it's the inability to reliably and efficiently produce actionable insights. At the next tier, you have diagnostic analytics, which offer some insight into the why of certain events, but not much in terms of actionability.
.gif)
They're easy to obtain but only provide hindsight, limiting their value. predictive and prescriptive analyticsīasic facts of an event, such as a sale, or raw key performance indicator (KPI) data, like a rep's quarterly upsell rate, are descriptive analytics. This oft-referenced model shows the value of the four basic types of analytics rising in correlation to the increasing difficulty of actualizing each type.
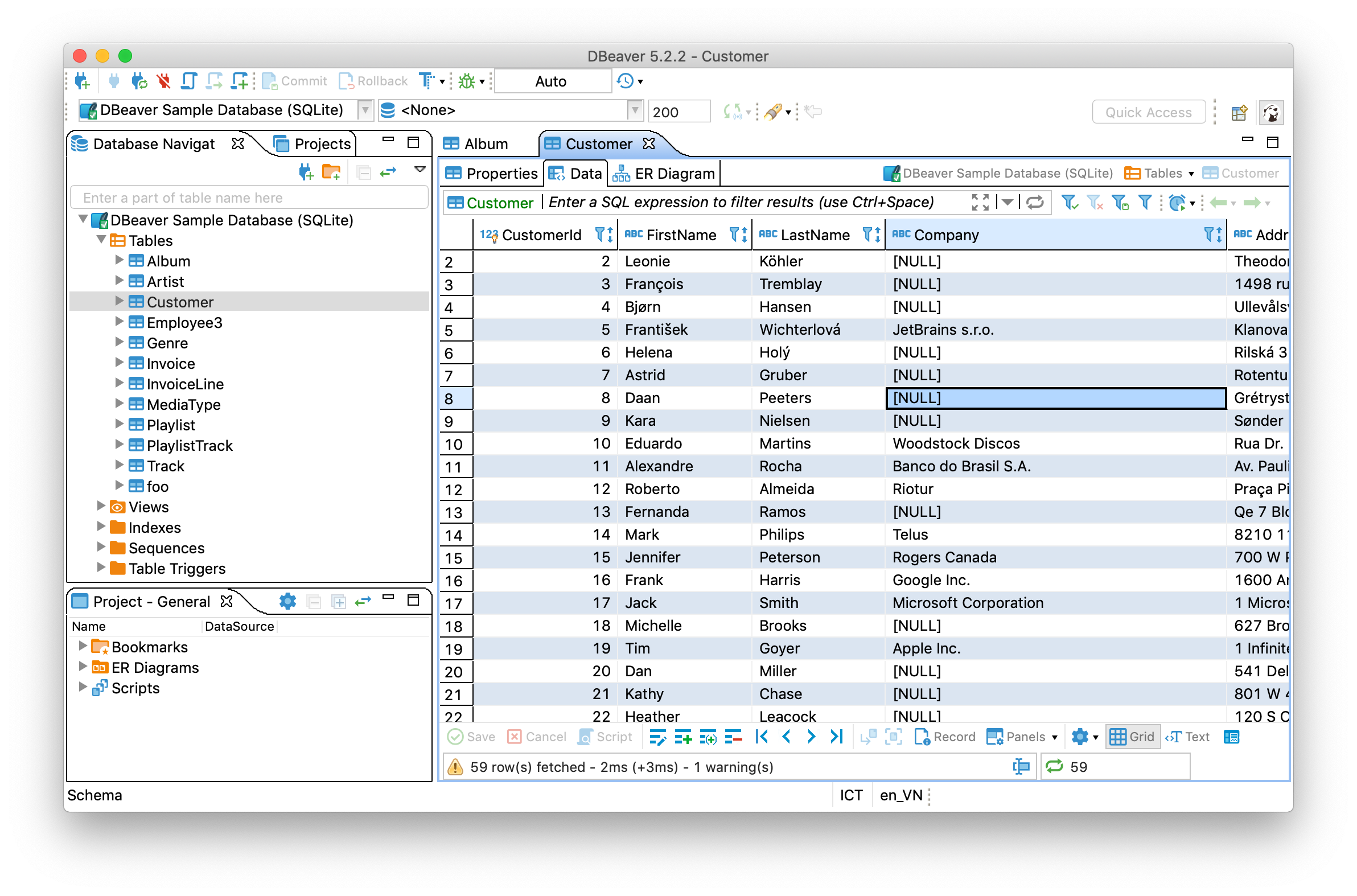
Gartner's analytics ascendancy model is useful in examining the gulf between traditional and AI-powered data analytics. They are simply limited in the analysis they can provide. It's not a matter of saying that more traditional data analytics are valueless, because that isn't true. But advanced analytics that run on AI or machine learning are nothing less than a whole new universe, in terms of the breadth and depth of possibilities they can help businesses realize. traditional analyticsĬomputerized data analysis certainly isn't new-organizations have been feeding tables of information into mainframes that filled up whole rooms since the late 1940s. AI- and ML-driven data analytics allow organizations to analyze, classify, and process many types of data from across a wide spectrum of sources, at whatever scale is required by the use case. Data analysis of information from specific segments of the business, like sales or marketing, or of particular domains like websites and apps, is also considered analytics.īy combining AI with advanced statistical analysis to create AI analytics, enterprise users may have the opportunity to leverage data like never before. A neural network that has more than three layers is considered to be a deep learning system.Īnalytics: Data analysis that clusters, segments, scores and predicts what scenarios are most likely to happen"-e.g., predictive analytics. Some ML systems mimic the input/output processes of the human brain, and are known as neural networks.
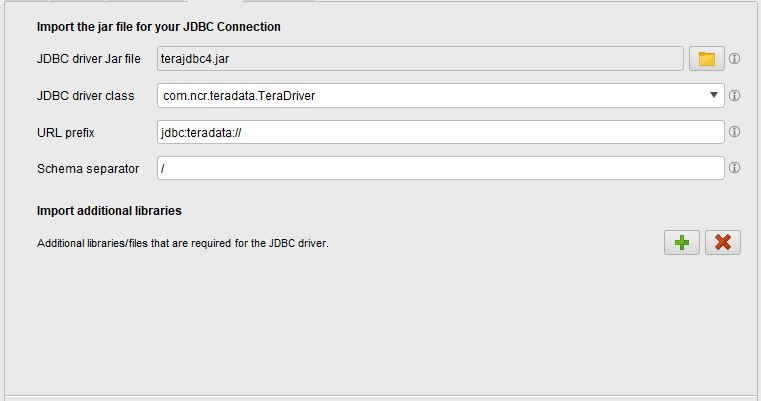
It uses more sophisticated algorithms that allow an automated system to "learn"-and improve its processes-based on the data it continuously retains. Machine learning: ML builds on the foundation of AI.
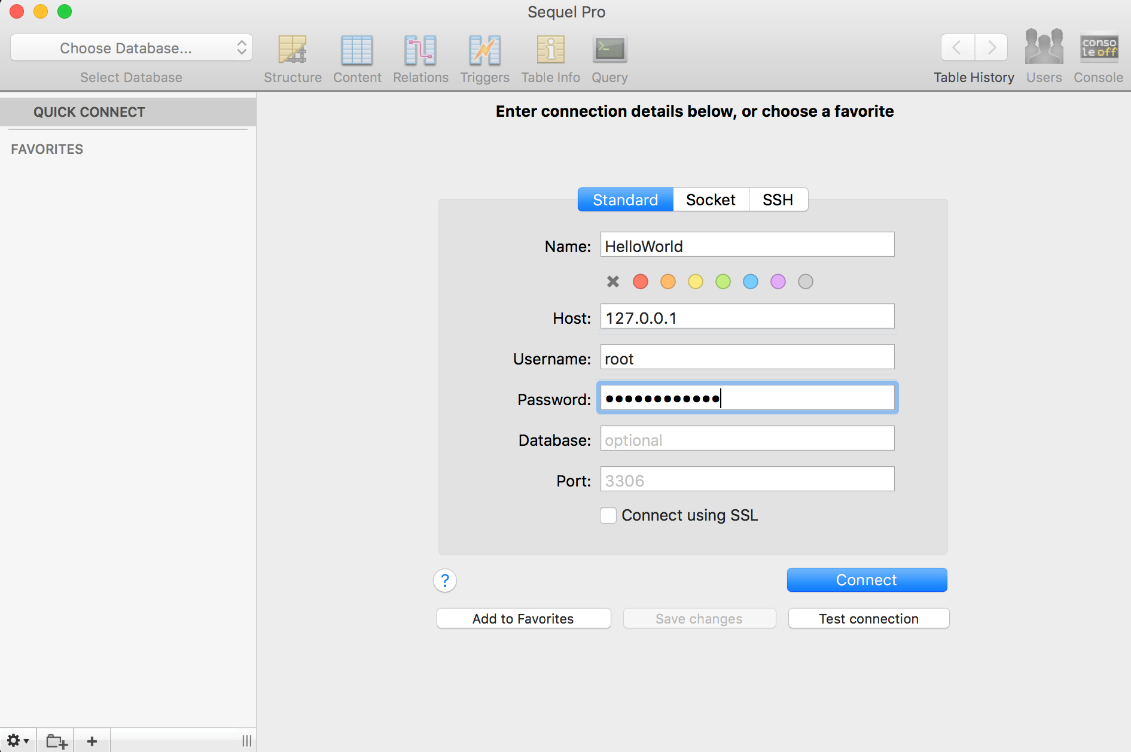
To better understand it, it's useful to define its key elements one by one.Īrtificial intelligence: This umbrella term describes the technologies and processes that allow advanced analysis and logic-based techniques to support and automate various decisions and actions, or to interpret events. AI data analytics involves the use of AI or ML techniques to accelerate data science and analysis projects and uncover valuable insights more efficiently.
